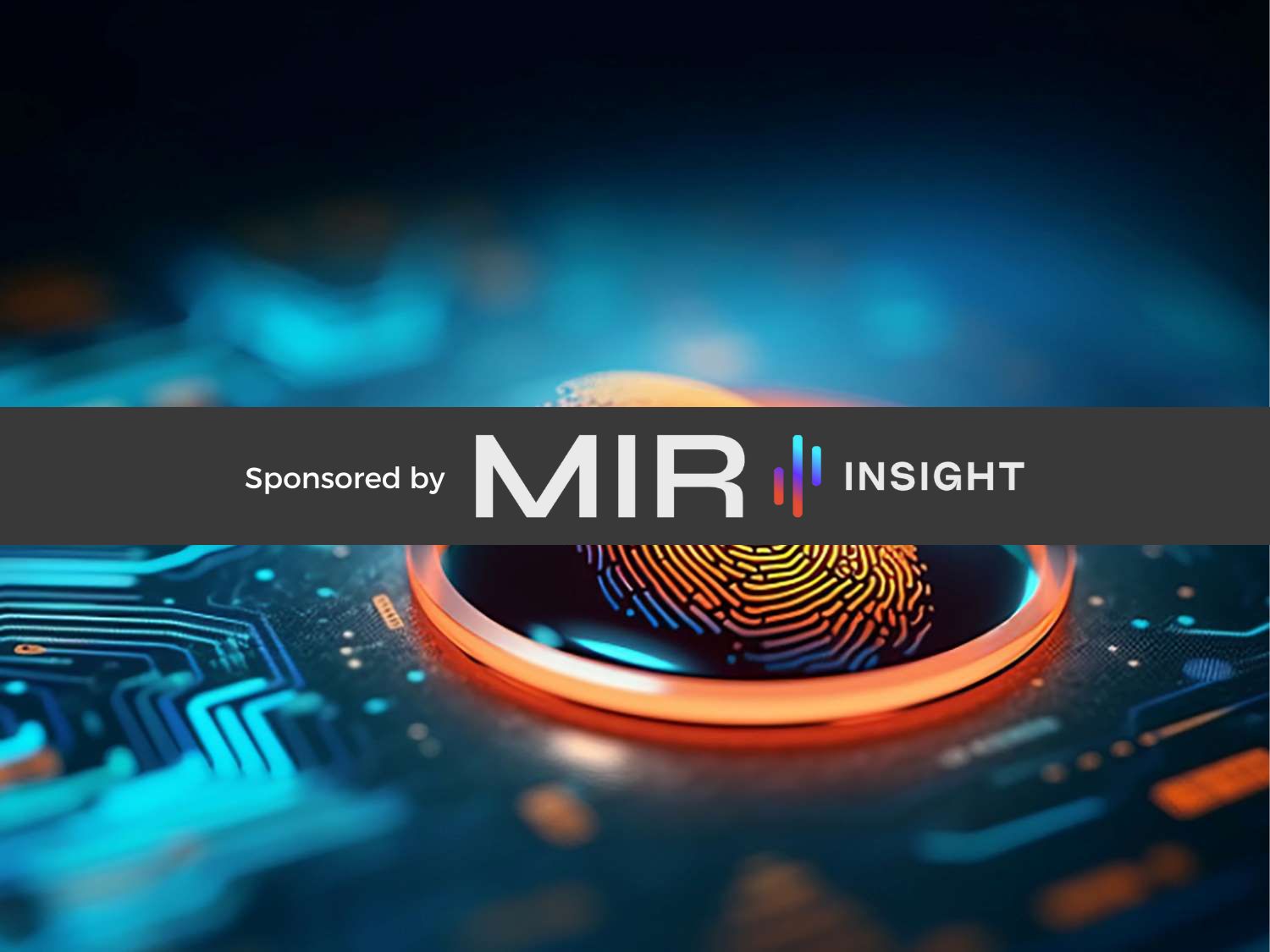
Overview
Title: Keynote: Using AI to combat price volatility and overproduction in the supply chain
Date: Wednesday 26 June 2024
Duration: 41 minutes
Have your say. Tweet and follow us @WTiNcomment
Summary
The textile industry faces significant challenges due to high price volatility and unpredictable demand. One of the primary culprits behind these issues is biased and inaccurate forecasting. In this presentation, Dr Bjol Frenkenberger, CEO and co-founder at MIR Insight, explores how advanced AI-driven real-world modelling can revolutionise forecasting for the textile supply chain, addressing these critical challenges head-on. A key highlight of the webinar will be an in-depth case study involving a leading yarn, fabrics, and garment producer, demonstrating how MIR Insight’s AI forecasting solution enabled this company to better anticipate market trends, optimise its supply chain and achieve substantial cost savings while reducing waste.
Speakers:
- Dr Bjol Frenkenberger, CEO & Co-Founder, MIR Insight
- Friedrich Weninger, CCO, MIR Insight
-
Transcript
This transcription has been AI generated and therefore may have some inaccuracies.
Using AI to combat price volatility and overproduction in the supply chain
The textile industry faces significant challenges due to high price volatility and unpredictable demand. One of the primary culprits behind these issues is biased and inaccurate forecasting. In this presentation, Dr Bjol Frenkenberger, CEO and co-founder at MIR Insight, explores how advanced AI-driven real-world modelling can revolutionise forecasting for the textile supply chain, addressing these critical challenges head-on. A key highlight of the webinar will be an in-depth case study involving a leading yarn, fabrics, and garment producer, demonstrating how MIR Insight’s AI forecasting solution enabled this company to better anticipate market trends, optimise its supply chain and achieve substantial cost savings while reducing waste.
Speaker 1: WTiN
Speaker 2: Dr Bjol Frenkenberger
Speaker 3: Friedrich WeningerSpeaker 1
Good morning. Good afternoon. Good evening, wherever you are in the world, you are very welcome to day two of innovate AI in textiles. I hope you had the opportunity to join us yesterday, we had five very full sessions, all of them, of course now available to stream where you are unable to attend any of them. All of them incredibly insightful. And the lineup for day two is no less stimulating. So here we are session one of day two. And to kick off, we will be having a keynote presentation from Dr. Bjol Frenkenberger. Someone I know very well, who is the CEO and co founder of MIR Insight, a AI forecasting company, I should say. I think on about three or four occasions yesterday, the application of AI in the area of forecasting was mentioned. But no one went any deeper into it. Everyone sees it as having huge potential in compacting the challenges of price volatility and overproduction in the supply chain. And Bjol will talk to us today about that. Bjol is also joined by his CE, CEO ce o o I do apologise for its failure, someone you may know very well, from his days, at Lenzing, and also currently the organiser of the global fibres Congress in Dornbirn Friedrich you're very welcome, Bjol. You're very welcome. And without any further ado, I shall hand over to you so you can share your presentation with us, Bjol.Speaker 2
I find your work. Brett's maybe we should briefly say who we are and why we're here. Um, so my name is Bill, Frank Miller, delighted to be talking to all of you. We funded mayor at the end of 2021. And I worked in my PhD at the University of Oxford, on the topic of uncertainty and how that influences business decisions, what negative impact it often has and the cost it has. And that's essentially what also fueled our interest in the topic and why we built this company because we know what great value you can unlock by using big data machine learning the new technologies to transform the textile industry into a more efficient and more sustainable player in the world, so to speak. And that's also our mission.Speaker 3
Thanks. Well, my name is Friedrich Weninger. And I have a 25 years industry experience and being the CEO of the Lenzing Group, which is biggest Manmade, cellulosic fibre producers and why I came across those guys was because as you might know, I mean, we continuously struggling on forecasting and you know, that stuff is very sentiment driven, not very structured. So you call up somebody in assists market is up or market is down. And this is how the how you get the information, how you should do forecasting. At the end of the day, though, learn to know those guys who are using big data and do it in a structured way. And those are using AI, machine machine learning. And so I was so interested to join that group. Thanks for letting me join the group.Speaker 2
Thank you for taking the time and joining us. Without further ado, let's go to the presentation. I'll bring it up on the screen. So there we go. The core of course of what we're doing relates to mark Get activity, external market activity, and how that influences you and your products, because we realise that many companies have very limited access and understanding of what's actually going on outside of your immediate circle of the people, you know, then this is what we mean by key warning indicators, is often these key risks, the turning points, these are difficult to estimate and difficult to capture. And so just to quickly understand what we're talking about today, so one is the problem cost of having limited market visibility, we will talk about how new technologies such as big data and machine learning, help us create essentially models of the real world, and how those models then reflect the future. We of course, touch upon the results also refer to a real world example with a producer of yarns garments and fabrics we're currently working with. And the process towards a more data driven company is what we will cover at the end. So to start off, of course, we know uncertainty is high, that has a real cost, I think everyone here knows this, we are in a period of high uncertainty, that is just being made worse by a number of trigger events happening now. In business terms, we know volatility is high. So this is polyester, for example. So if we look at polyester prices, we know these were swaying back and forth around 50%. Plus minus. And we also know, on the demand side, so here we're looking at apparel imports to the US. So demand side is equally volatile, with 50% swings in more in the last few years. And all of this fits, I think, you know, this also quite well, it creates a real costs. Maybe you can say a few words like for this example, Howard, we can understand these numbers.Speaker 3
Yeah, I mean, just to summarise, I mean, what's the implications, and if you are, say you are, you don't see the tipping points, right? If it's going down, or if it's going up, I mean, firstly, of course, you see, after a couple months, say two to three months a big price erosion, erosion, usually, when the market goes down. So you're sitting on stocks with high prices, then of course, the next step is, stock levels still high, so you start selling off. And most of the time, you are reducing the production. So this is reduced less output. So you can imagine that the fixed cost coverage is not given any more. And then, of course, you see all the other financing costs, you have to finance the working capital. And what you're also doing, this is just a sight to the customer. But if you see the sight of the suppliers, you bought the raw material at the wrong time. So and sitting, maybe then on stocks, so this all in all, creates, I'd say a five, minimum fives, six to 8% of turnover, which is normally lost. If you're doing if you're having a bad forecasting safe for a six to 12 months base. And this is why I got so really excited about the system to just do it on a structured way. So really, you can believe as losses, what percent revenue is absolute minimum? We have examples which move up to 8%.Speaker 2
Yeah, and that's, that's not pleasant for anyone in the industry. And we know of course, this is an example but there are a number of companies that have suffered Unfortunately, these losses in the last few years.Speaker 3
Yeah, and I also would add, would like to add fuel, if it comes to you European Commission European Union now the textile strategy of a so requires reporting on the resource management on the efficiency and we are urged to show that we are getting better and not less efficient. And so this is also something which we really pop up in the years to come that we have to do reporting how we get better in resource management.Speaker 2
Yeah, that's that's a totally agree with that of And what we see the problem usually in the industry, which links directly to what you just said, is that we have this problem of overproduction. And I think you're aware of this, but I'm just pointing it out how we see it, of course, that leads to waste and costs. And the way it usually looks as of course, that if you're at the back of the chain, and you're producing yarns, for example, which then goes to further steps and ends up at the end consumer, you do not really have the insight into what the end consumer is doing. And there is a lack of information exchange, which creates uncertainty creates the overproduction and also creates waste, and costs. And if we look at, for example, the polyester where that's coming from, which is of course, oil, here, we have high volatility, which affects profit margins. So here we have, you know, the one side is the oil side, that goes into the raw material, the base material and for the polyester. And again, here also you have a demand side, which influences the prices, of course. And there's again, no information exchange between the input factor side and also the demand side. And that creates uncertainty around prices. And, you know, what we do here is that we say, it's really unfortunate that there's billions of data available in the world. But it's so difficult for producers and for companies in the supply chain to use that data in a better way to forecast their own products, the prices they care about, and the market state care about. And so what we do on the one hand is we put together all these billions of data for you, we clean them, we make them ready, we purchase them, we then identify what really matters in that data, what is going to be an important influence factor for you or for your business. And based on this selection process, we then forecast demand price developments, market developments. So just so you understand how that works on a more technical basis. So once again, here you have the billions of data that we put together, across macroeconomic data, financial data, global risk, climate data, etc. We identify real world factors that, you know, have been proven to influence the last 10 years, your demand your your prices, we then use this to create forecasts that are up to 80% 90% more accurate than if you forecast without those factors. And of course, we output it also, we visualise it for you. And you can also make up your mind by examining those outputs. While we also offer now, API integrations with your preferred ERP systems, such as data txt, which is very prominent also in the textile space, of course, as we all know, and who is our partners? Well, I want to maybe briefly touch upon how we actually show this to you how you can actually look at the future. So one way is to look at an absolute value, such as here, you'll see the data points. So you simply know, the next six months are going to be somewhere between in this example 86 and 95. Or you can look at the trend. And here the question is, are the next six months going to be more positive, more stronger? Are they going to be outside of the barrier? You'll see here? There's a dotted barrier, the lower and the upper barrier? And the question is, is going to be outside of this barrier or below. So this is a different kind of question. And we offer these different ways of visualisation. Because we know these are different ways in which people like to evaluate the future. So to give you a concrete example of what we're doing, this is currently also what we show together with WTI N. We are looking here at us import trends. And we see firstly the quality improvement, which is the improvement you have in the forecast thanks to the market factors we identified out of the billions of data we have on our side. You see here, first, the forecast and the historical data, the forecast and all of green and historical data and dark blue and share you see the different factors that were selected. For example, on number one and two, we see factors directly related to consumption. So how much do people consume Number Two export of nitrous is, of course, also directly related to textiles, Germany, a big exporter of textiles. And this gives you an idea of future imports into the US market. We see how important these are 100% 76% weighting. And the lag gives you an information on how much time difference there is in one data set. If there happens something one day decide when does it affect us? Imports, for example, you can see, there's a number of days that you can select this leg to can make it visible. And the selection is purely automated. So we use this algorithmic logic we have developed to find the influence factors. And that can be done for any product, you're interested in your own products, or also general market data, of course prices as well. And very important here also the quality improvement you see. So how much better is this forecast actually, in comparison to classic way of forecasting, and how does the model learn over time because the machine learning model learns continuously and it gets better and better, the more data it has, until it really also outperforms a human user. So lastly, goes to show that there's similar data sets available, of course, that you can purchase an axis. And this is what we also present currently with WTI n, where you see an example of these external data sets that we also forecast across different apparel, and categories from from wool to cotton, to inventories and shipments in the US itself, as well as us textile production. But again, this is an example of eight different forecasts. And there are many, many orders from fibre prices, etc. That we also offer. Because we understand of course, the prices are very important for decision making, as well as the demand side. And here, you see that this is done in the same principle, we're also using these external factors to forecast the future trend of these various import data. Okay, let me go on, for instance, there may be anything you would like to add? No,Speaker 3
I'm fine. I mean, there's a lot said already. But I'd like to also maybe dimension is that we are working the supply chain and the textile industry is really long. So it's a bit foggy around the place, the more upstream you are like, we as Lansing as a pirate newspaper, or as a polyester producer, of course, there's really not a good visibility. And so we our vision is now to get more and more and more people in the Textile Supply Chain. Because then the, the accuracy of the forecasting is getting better and better and better. And we are very, very well on our wish and so to say. So we have them from the fibre producers side from the textile producers side to the printing and to the apparel making. So that forms as a very good visibility. And and this is the final outcome for you all guys watching us now that you're getting also more visibility on forecasting.Speaker 2
Yeah, that's a very good point for it's and one thing is that, of course are models, the data we use is more and more specialised to the supply chain to textiles in particular models as well become fine tuned because we are training throughout the supply chain. And as a sort of third step, we would also offer access to a premium data pool that is opt in based. So if you wanted you can be part of it, you get access to it. If you are not interested, you do not need to take part in the system. But that is part of our offering as well. So one more point I wanted to make regarding the trend forecast, because we know that cotton prices are of great interest. So we hear data trend forecast of cotton. And you see these detection probabilities. And the point is you have a 50% detection probability you capture on 50% of changes, but you captured them very accurately with usually 90% accuracy. And that relates to the factors you see here that we made visible. We did another version of this where we worked with With factors that were selected by the customer, and here you see we adapted the factors. So you see a selection of civilian labour force in the Midwest global rapeseed oil, which is also an agricultural product. And workman moves together with cotton, and also different data on climate Storms in Africa, floods in Africa. And this is the kind of flexibility we can offer. And then you can see how these different metrics change, do you have more detection probability, so the forecast is going to change, actually, we what we saw is that the forecast was in this example the same so you'll see a slight tendency towards the negative side here, so that it will slightly decrease at the end of the year. But we have some changes in the detection probability. So as you change the types of input factors you used, you had a higher detection probability of a change. And the, you know, as I said, the accuracy is always very high here. So it's around 90%, that you're capturing the right direction. But you don't capture all the changes, because not all the changes are related to these factors. There are other things people, you know, set up the prices, people change their mind, there's a lot of these other things that also play a role. And so these these things are, of course, not captured. But we show you exactly what we capture, and what is going to be the expected outcome of them. The similar to this, I want to touch upon when we talk about accuracy. And this is really important in forecasting. So I also want to explain to you actually why we know performance and why we know that we can trust the performance. So this is, for example, where we worked with a producer fabrics. And here you can see the history of the last five years. And all the green lines are where we correctly predicted a change. So you see this was very, very correct, we didn't capture all the changes you see here is some changes in between. But when we indicated a change, we were very correct. So which is somewhere around 90%, in this example. And so what you then get as an output is, of course, we can tell you, okay, the fabric orders in the next six months, are most likely going to be within or below the indicated corridor here. And that is already very useful. Because there's a benefit to this. Of course, if we remember this example, from the beginning, there's a real cost to getting it wrong, right? It's just somewhere minimum, for instance, we said 4% of revenue. And if we, if we look at the customer themselves in the size they had, the problem was really that there was no good order forecast, the changes in the market, make purchasing, production planning, etc, a real challenge. And so when we saw that we could capture an accuracy of 80 to 90%, in six months for orders. So it was all about orders and demand, which is an increase in accuracy by around 50 to 60%. Which is similar to a loss of either the front 2.5 million. So we're looking at 100 million revenue company here. And these were for reference all the different things we did. So you'll see we looked at garments, fabrics, different yarn types, and we had an average accuracy somewhere around 80 ish percent for the next six months 80 to 89%. And we did different types of forecasts, which we also show here on the right.Speaker 3
You're Amana at Samsung. Yes, why we are so focused on a six to 12 month basis. And not just on not just on three year five for 10 years. Because as you all know, you're from the industry. If I know the tipping points, six months ahead, I can manoeuvre even four months ahead, I can manoeuvre, I can really be more aggressive on pricing and sell off stocks, for less aggressive to really wait a little bit and see how the market develops. And this is such an important information to really change, let's say the modus, I'd say the more the inner company, how to act on the market, for instance, and and this is six months, four months, six months or eight months is absolutely good in order to achieve good results in terms of performance.Speaker 2
Absolutely. And and we do use the same logic, the same window also for general market data because we know you know a lot of companies are exporting to certain markets or price data, which we'll also say is general market data. So there you also of course are interested in the inflection points usually So that are happening within the next six months, or six to 12 months. And the forecast we produced this also always refreshed every month. So we can even refresh more frequently. But we know that a lot of customers prefer monthly, refreshing after forecasting. In total, actually, there's around 2 billion datasets that we can access. And we can also do forecasts on for for whatever you're interested in. But of course, for practical reasons, I think it's usually maybe around 1000, or 2000 datasets that are really of interest to most users in the textile space. And we use the rest to forecast these key datasets that we have. Just So to summarise, I mean, we talked about it, but what we can really also focus is on your own data. So just to make it clear, and this is what we used to do now, and the yarn space and the garment space, also with cotton products and fibres. And there we look at the orders, the deliveries, the regional activity, the product categories, and also look into cost factors such as the purchase prices for particular synthetic materials, and other raw materials such as cotton as what I showed you in the beginning. The process here, how we work as sample, we we meet you in a first meeting, we do a workshop with you. So you understand what is the best forecasting setup, we do a testing where we're saying, okay, don't send us all your data, send us some of your data, and we simulate 2023 with you. So you get a feeling as to how well the forecast performs. And all we need is an actual file. So not a lot of implementation necessary here. And then we go into the real forecasting process with you where we, we work on those billions of data, we make them all accessible for you, and you can then use them. With us with our support, to focus better and more accurately, according to what happens in the world. We are not leaving you alone. And we're almost involved as well. We offer this via our AI platform. And also together with WT N, of course. And if required, we can also go all the way to an implementation with your ERP systems. We already introduced ourselves, I simply want to lastly also introduce to others because we are of course a new technology company. So it's always good who know you're working with on the tech side we have with us no no who is overseeing our engineering team. 12 years of experience former methyl limpid winner, nominated us programme of the year. And we also have Jonas Faulkner who is a PhD in machine learning, he oversees our data team, who is somebody with more than seven years of applied AI experience with a focus on supply chain and logistics in particular. So frets of there's something else, please mention now because I think we're gonna wrap up.Speaker 3
I think it's basically all set. Okay.Speaker 2
So so to just sum it up, I mean, we see there's a lot of volatility in the space. I think everyone here knows that. There's a real cost attached to it. We did also an estimate on the whole industry. Actually, we look only at the forecasting alone, we know somewhere between 100 to 300 billion wasted each year in textiles, which is around 1% of the global greenhouse gas emissions as well. So that's why we know it has a real impact the real cost ratio forecasting, our team of course, is ready for you. We bring you new technology to tackle this challenge. And all you need is an Excel so get in touch and we would be very happy of course to work with you and to explore what can really bring value to your organisation and your products. Thank you very much.Speaker 3
Again,Speaker 1
we all threats. Thank you so much. Something that is so complex, billions of datasets, you make it sound very clear and simple as to what this technology is able to achieve. And we've received a number of questions for you, which I'd like to throw at you now. The first one says that the supply chain is quite an unpredictable place. Yes, that's true. How can AI modelling stay ahead of volatile or tumultuous changes to the supply chain to ensure that it always is always accurate? I think what we're referring to here is a kind of a black swan event, and how you can react to that, and how quickly you can react to that perhaps?Speaker 2
That's a very good, very good point. And I might jump back into the presentation, we have an additional slide here. Of course, the user is the question is correct from from the visitor of the webinar, we are not able to forecast black swan events, because that is the definition of a black swan event that is not forecast. However, you can see even in things such as the large scale invasion of Ukraine, that you can adapt much more quickly. With this kind of forecasting logic, then the customers on sales budget. So what we see here in green is the sales budget, the actual demand is in blue, you see the forecasts that we had, at that point in time in red. And at the end, of course, if you look at the period around September 22, there is a significant gap between the sales budget and the actual demand. But this gap is much lower in our forecasts. And this is part of the value, of course, that if you have an adaptive forecast that's based on indicators that have influenced the demand and influence the prices, then you can anticipate these changes better, and you can adapt to them more quickly. And that is very, very important, of course, to say,Speaker 3
I think they're always singular events. Yeah, I remember back to pushing him up supply chain disruption, Suez Canal supply chain, disruption, never happened that went on forever, so to say, and I quote, so when this is why the system is very adaptive, and has historical data is in hand and learns out of historical data, and adapts much faster than the human being, so to say, and this is what the graph shows here. I mean, we were pretty much on the line, there is still Korean War, whereas human beings with the sales budget, still didn't really know what to do at the end, if you see that green line.Speaker 1
Some more questions coming in here for you. Next question, what AI techniques are most effective in forecasting demand and managing price volatility in the industry? That are how much you want to reveal about your techniques?Speaker 2
That's a very good question. I mean, we can of course, touch upon what is the difference between what we're doing and for example, chapter 80 is doing our approach is as different from chapter 80 as a chainsaw from a Swiss army knife. So if you want to go into the forest and fell a tree, you need a chainsaw and a Swiss army knife and price demand forecasting is the chainsaw approach. So you need a very specialised tool. And the chapter beauty uses a more all rounder approach, right? So where you can do a bit of everything. And your focus is very different. And your data gets mixed with the data of other people. And so you lose a lot of the control. And we use an approach that trains models that are specific to the problem with data that is specifically selected for the problem. So that the model can is really like like a specialist and solves what it should solve instead of fantasising or coming up with things that do not make a lot of sense.Speaker 1
That's a follow up question from the from the same delegate. How do you validate the accuracy and reliability of your AI forecasting models.Speaker 2
Yeah, they would maybe, again, refer to the initial presentation. As we certainly all just go back to this one slide. The verification is, of course, a more complex process than I share now. But in large terms, in general terms, it is as it's shown here. So what the machine learning does is it predicts throughout time throughout the last 10 years, as if it doesn't know that it is in the past. So the model actually does know it, of course, we know that it's in the past. And then it compares with what has actually happened, whether its own predictions were correct. And this is how you fine tune the model. And this is also what this graph shows. So the thick green lines here, show when we were correct. And also the the sort of dashed green lines, the thick green lines show when the models said there would be a positive development in trend and the dashed lines when there was a prediction that there would be a negative development and trend. And so you see that the one time it was wrong, somewhere around COVID Was that it did not expect the trend to reverse immediately. But then it was correct when it predicted the continuation of the trend in 2122, and so on and accurately then again, captured negative trend, somewhere around 20 to 23.Speaker 1
Thank you, Bill, question here for Fritz, with your textile industry experience, we'd love to know how, how do you foresee AI forecasting? Changing the textile industry? That's quite a big question. But I'm sure there are some themes that you can share with us.Speaker 3
I mean, I see AI and machine learning very much as a support as a support for the for the for the forecasting. So far, we just rely on on, let's say, on the sentiments of the customers of the customer of the customers, etc. And so we get be put in, in our forecasting, a lot of I'd say, a lot of gut gut feeling, right. So I did it quite quite often during my time as a CEO. And, and this is now giving me another opinion, where I can really be more, let's say challenge in a way. And so I say really very, very often, because I'm in a supervisory board, subcommittees. And so yeah, we have to improve the SOP process. But the SNMP process will not get better if you don't get better forecasting. And I think this is an additional support of AI and machine learning that the the forecast going into the system will be more accurate. And this is what we aim to, of course, the optimum would be that everybody in the supply chain will participate. And then it's better for everyone, that's for sure. Because we have more stabilising stock levels during this in the whole supply chain, which is not the case right now. It's quite a disaster, I must say, you know it also mark from your interviews and your guys, you know, in the textile industry, it's it's not it's not efficient. It's very long and very fragmented, small and medium sized companies. And that's the issue. I see a lot a lot of support a lot of support by AI and structured approach.Speaker 1
Thank you for its and on that positive note, we do have to draw this session to a close. And I thank you both for your time this afternoon. As always, most stimulating and interesting what you're doing. And I've no doubt that some of our delegates today will be reaching out to find out more about the service for you in the audience. Please join us again shortly. We'll be back at 1pm UTC for our next session, which is a present Asian by Raj pactor, who is founder and CEO of Chem AI, they he will be exploring how AI is changing the chemical industry and the discovery of chemicals. We'll see you back in 20 minutes or so.